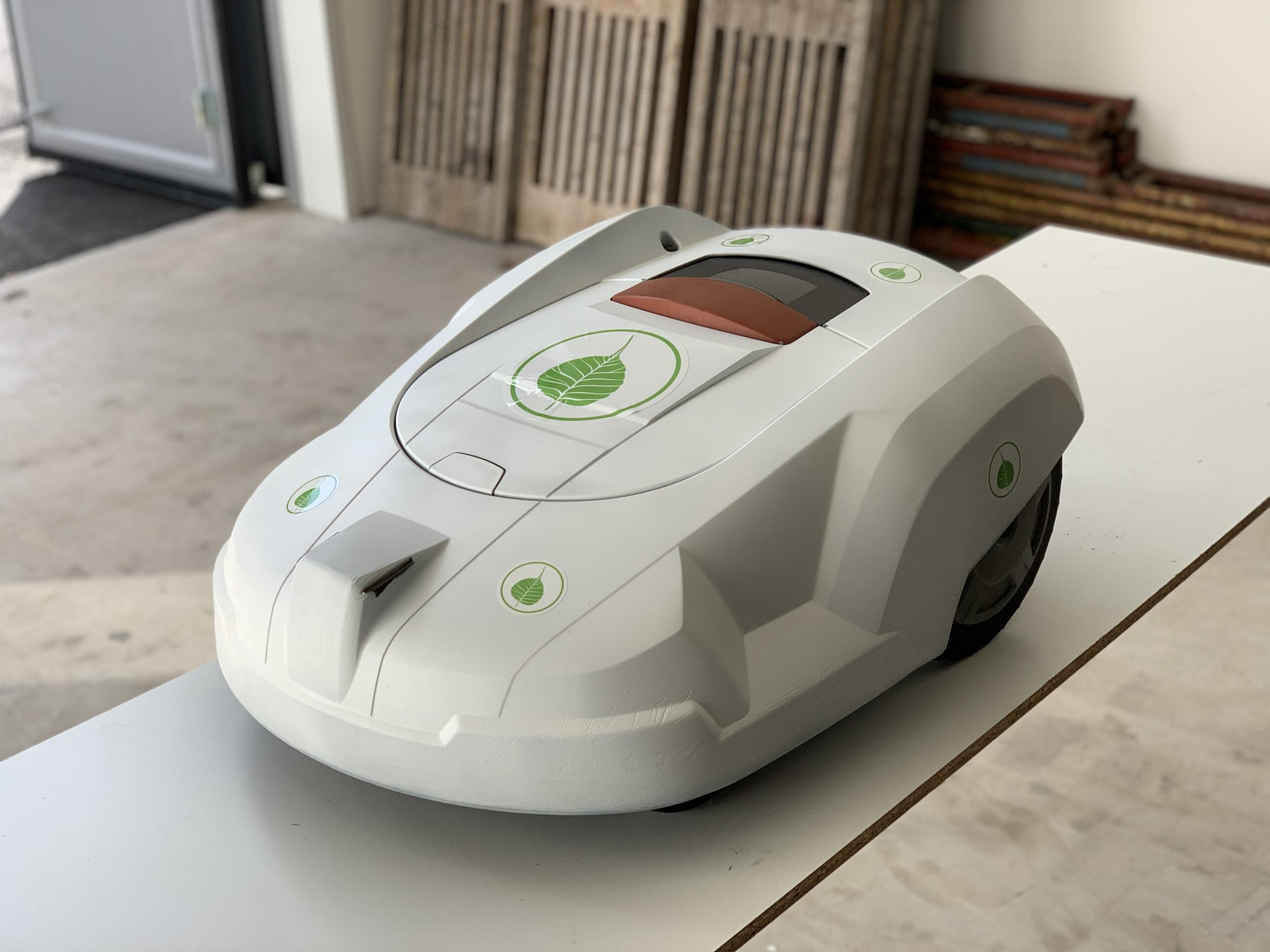
The internet of things (IoT) is not just about connecting devices, but about making those devices smarter. This conviction underpins the vision of Jon Lindén, CEO of Ekkono. The Swedish start-up makes machine learning software that enables even tiny products to adapt to their local environment.
The implications are far-reaching, with the potential to completely transform business models. Energy efficiency is often associated with doing less, but machine learning allows companies to improve products out in the field. While historically a product was at its best when it left the factory, in future that product will only get better over time.

Founded in 2016, Ekkono is working with big, established energy players like ABB, Siemens Energy and Volvo to take the energy transition to the next level. In conversation with Energy Monitor, Lindén talks about his technology plans, headaches, and Tesla as a source of inspiration.
How much of your work centres on the energy transition? What are you most excited about in that context?
Starting a company demands a significant effort. From the beginning, it was obvious everyone at Ekkono could gather around sustainability. You can optimise a gas or wind turbine so it runs at its very best or you can do smart grids, but what I find most exciting is consumption. That is where technology can make a big difference.
Technologies are just tools, however. We are a machine learning company [using computer algorithms that learn from data and improve with experience – a form of artificial intelligence (AI) – to optimise a product’s operation]. We do not have the domain expertise our customers have. We are a tool to help them, just like a monkey wrench.
How can machine learning transform energy efficiency?
OEMs [original equipment manufacturers] have been optimising product design for decades. The next big leap forward is to operate every individual unit at its best. In other words, we need mass customisation.
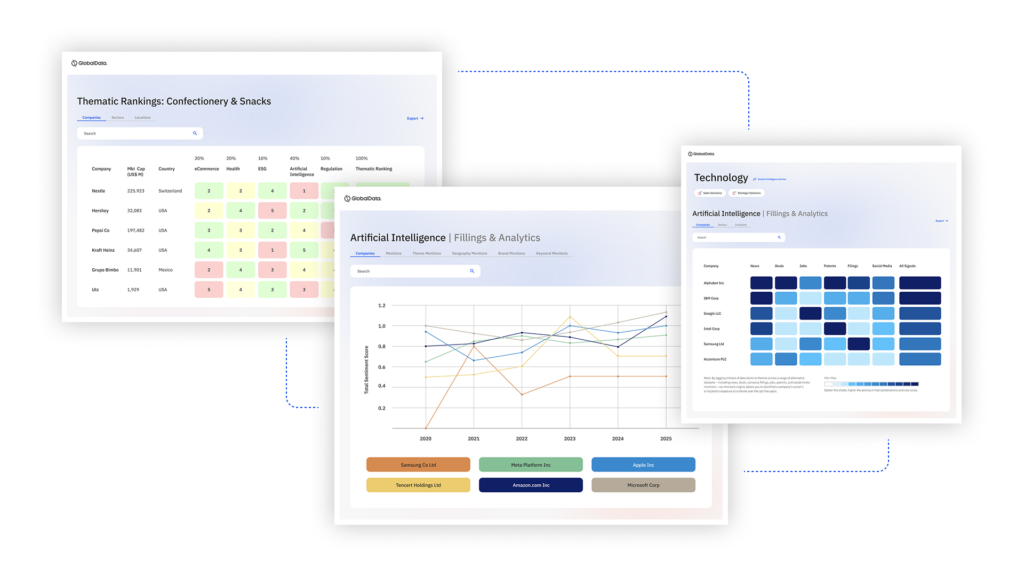
US Tariffs are shifting - will you react or anticipate?
Don’t let policy changes catch you off guard. Stay proactive with real-time data and expert analysis.
By GlobalDataTake an air conditioning unit. You want to understand, for example, what environment it operates in, its purpose, the preferences of the people who run it, how the room it sits in is used and whether it is winter or summer. This is where machine learning is awesome. It can take a lot of different input values and learn from that data rather than having an engineer go in and tweak the unit every now and again.
If you optimise how every individual unit is used and multiply that by the millions of air conditioners sold every year, you can really have an impact.
What is the biggest challenge you are coming up against?
The rate at which companies are really implementing these new technologies is a challenge. There are lots of pilots, but to get to where you roll out at scale – which is where the benefit is – it is still way too slow. We do not have enough testimonials and real RoIs [return on investments].
As an entrepreneur, uptake always seems too slow, but in this case I have been surprised at how slowly IoT has been adopted. I can think of a number of reasons. One, there is often an installed product base which is working fine. On top of that, historically most OEMs do not want to upgrade products out in the field because that is not where they make money. They make money by selling new products to existing customers.
From a sustainability perspective, upgrades to an existing fleet make a lot of sense, but I underestimated the time it takes OEMs to upgrade or replace products.
A second reason for the slow roll-out is that 80% of people are followers. You need to find the 20% that are ready to take the lead and prove something works.
What interest do you see in a shift from selling products to services, from hardware to software?
The interest is huge, but adoption is not as big. We are proposing a strategic change that impacts a whole business.
Tesla has proven that even a capital-intensive industry like the automotive sector can be turned upside down by a start-up.
No CEO can ignore sustainability. They understand they do not have a future if it is not part of their priorities. Most also understand that if they do not disrupt themselves, someone else will do it. Tesla has proven that even a capital-intensive industry like the automotive sector can be turned upside down by a start-up.
Historically, a product was at its best when it left the factory. That is not acceptable for the future. The product that comes out starts to learn: how it is used, for what it is used, and how to optimise use for that purpose. It gets better over time, and then you have to rethink about what you charge for. How can you replace a product that is better than the one that left your factory?
You are work with some big names, including ABB, Siemens and Volvo. What kind of business model could work for OEMs such as these in future?
The low-hanging fruit has to do with maintenance. Customers believe you connect things and you collect data, that is IoT, but that is not its value. The value is in extending the relationship with your products after they leave the factory. You know the status of those products so you can provide a different kind of maintenance service. You know how they are being used so you can charge for use, rather than the product, and you can help customers upgrade their products.
We see opportunity, especially in industrial end-use appliances. Take buses. Instead of buying buses and having ten spare if one breaks, you buy a guarantee that you have the buses you need for your miles. If Volvo can sell buses as a service and maintain them more efficiently than the customer, it can make more money.
Most machines and vehicles already have sensors. The question is what you do with all that sensor data. It boils down to how you can combine greater efficiency with a business case. You can look at how to optimise battery consumption on every bus, but for whom is that an incremental value you can charge for? We tend to forget too, that sometimes you have to invest now for a pay-off later.
Take smart battery management. If you know with more precision a battery’s remaining range, this does not mean you can charge for that but it does make the product better. Monitoring exactly how a battery is used – and deteriorates – in a specific car might enable you to charge it in a more efficient way, and extend its life. Somewhere down the line that will translate into either a superior product, or a separate feature you can charge for.
Everyone is collecting data. How do you know what to measure and analyse? And how much of this can be left to machines?
You do not let data scientists make the decisions! You rely on domain [content] experts [at the OEM] to shape and interpret data.
Machine learning favours those with the best domain expertise. Companies like Husqvarna [a Swedish lawnmower maker] have been around for 330 years. [With machine learning] they can really sell on the back of the fact they know how to take care of your garden. Someone else can probably design a lawnmower that is as pretty, efficient and cheaper, but if it has been developed by people who know less about how to mow your lawn, it is a lesser product.
You can use machine learning, sensors and digitalisation to build the know-how in a company like Husqvarna into its hardware. Its product will be better from the beginning because it knows what challenges lawnmowers run into and is designed to deal with them.
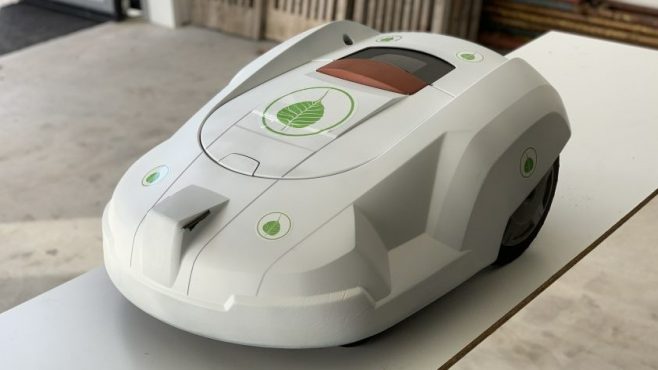
So, in future, products will differentiate themselves by their software, not their hardware?
The hardware is never going to be better than when it leaves the factory. The part you can enhance is that it gets better and smarter features.
Look at Tesla: it sold an upgraded feature allowing customers to buy a parking assistant. The car already had all the necessary sensors, but you paid an extra fee for the parking assistant because it was new. When it didn’t work in Sweden because the climate was too cold, Tesla uploaded new software that pre-heated the battery. The company enhanced the product out in the field.
How big a concern is cybersecurity?
Powerful tech always comes with a risk. We have to balance the opportunity of using technology – in this case, that billions of devices could save energy and help meet our climate goals – with the risk of people using it for bad purposes.
[Keep up with Energy Monitor: Subscribe to our weekly newsletter]
We carry around a much bigger risk in this [smartphone]. In IoT, there are risks, but it is easier to maintain strong security around a connected product than a connected person.
That said, if our phone is hacked we will typically realise it because we use it all the time, but I do not check [the security of] my fridge all the time. We probably feel a bit unsafe because we do not actively monitor these products – but generally, things are safer than people.
What kind of AI or algorithms do you use, and how is this different to other AI companies?
Everyone is talking about deep learning [a subset of machine learning with algorithms inspired by the brain that learn unsupervised from unlabelled data]. It is extremely suitable for some things, like image recognition and natural voice translation, but not for everything. The whole nature of deep learning means it is not interpretable. You cannot look at the algorithm and figure out how it reached its conclusion. You have to trust it.
We do not do deep learning. Instead, we have implemented a number of different machine learning techniques, including simple ones like linear regression [a machine learning algorithm based on supervised learning with labelled data]. Some of these you can read like a mathematical equation. If the algorithm changes a setting on a machine, you can understand why.
Our strength is that we can run on very small microprocessors, making it easier to do retrofits. If you retrofit, it will be onto older, less powerful processors. You have to make your technology as energy efficient as possible. That is why we have someone with a PhD who is actually specialised in energy-efficient machine learning algorithms.
Looking forward, I think edge computing [computing done at or near the source of the data i.e. on devices on the 'edge' of the digital system] makes a lot of sense because we will already have computational power in things. We can use that processing capacity instead of it idling and turning to the cloud or making extensive use of [energy-intensive] servers instead. It makes sense to do the first level of intelligence at the edge.
What is your measure of success?
The transition to automated, connected products is via machine learning. What we provide will be used in all connected devices. It is a gigantic opportunity, but the way I will measure success is if I can walk through town and point and say 'there is Ekkono in that and that and that’. If the truck passing by, the air conditioner on the wall and the lawnmower in the garden are all a bit more energy efficient and do their job in the best possible way, then I can say we have been successful.