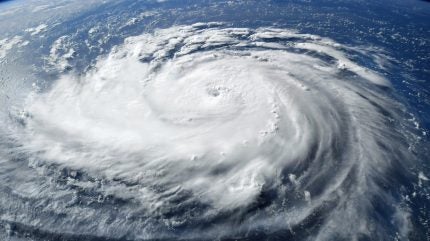
Climate change is set to disrupt our way of life as we know it, bringing with it unprecedented heatwaves, rising sea levels and more severe storms. While it can be predicted what land will be submerged underwater in the coming years, bouts of drought and flooding have been more difficult to predict with accuracy.
To try and solve this blind spot, researchers at Google have developed NeuralGCM, a machine learning-based approach aiming to simulate the Earth’s atmosphere.
Intending to eventually scale up to a full climate model modelling the oceans and the carbon cycle too, NeuralGCM combines traditional physics-based weather models with machine-learning techniques to generate very accurate two-to-fifteen-day weather forecasts alongside the potential for much longer timescales.
According to Stephan Hoyer, a senior staff software engineer at Google Research who has been working on the project: “NeuralGCM is one of the first AI models to show promise for this longer-term climate projection,” resulting in benefits for governments, infrastructure providers and businesses alike.
Combining AI with climate models
While incomplete physical understandings of processes such as cloud formation limit traditional climate models, NeuralGCM’s ability to learn from historical data facilitates a more detailed understanding of the processes culminating in weather events.
“From a technical perspective, we combine aspects of AI with traditional numerical simulations that have been used for many decades for weather forecasting and climate modelling,” explains Hoyer.
How well do you really know your competitors?
Access the most comprehensive Company Profiles on the market, powered by GlobalData. Save hours of research. Gain competitive edge.
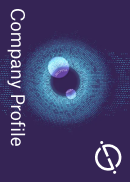
Thank you!
Your download email will arrive shortly
Not ready to buy yet? Download a free sample
We are confident about the unique quality of our Company Profiles. However, we want you to make the most beneficial decision for your business, so we offer a free sample that you can download by submitting the below form
By GlobalDataNeuralGCM works similarly to traditional models by dividing the earth’s atmosphere into cubes and calculating large-scale weather processes. However, instead of relying on estimated parameterisation, it uses neural networks to learn the physics of these events. This means the model can account for the effect of small-scale features on the global weather and climate model.
With these insights, the model can work with longer time scales to predict weather patterns, and it should be possible for the model to predict if next year will be drier than average, or what trends will be over the coming decades as climate change intensifies.
How NeuralGCM differs from other models
While many tech companies are experimenting with using AI for weather forecasting, such as Nvidia’s recently announced StormCast, Hoyer explains that due to NeuralGCM long-term focus, Google Research’s model addresses a different issue.
In contrast to similar approaches that have been successful for weather forecasts, “the real difference”, according to Hoyer, is that Google has been able to train the model on a lot of historical data which allows atmospheric models to be corrected to simulate weather phenomena forming below 100km such as fog, cloud and rain.
Hoyer also emphasises that NeuralGCM is significantly faster than existing climate models, up to 100,000 times so than some existing climate atmospheric models of similar accuracy, he says.
“That’s a transformative speed difference,” adds Hoyer. “It is going to allow for a lot of experimentation and sort of exploration by scientists. It’s like taking the supercomputer onto a laptop.”
Developing a climate model compatible with AI
Central to the success of NeuralGCM was Google Research’s decision to rewrite the numerical solver from scratch in Google’s machine learning framework JAX.
Hoyer explains that one of the challenges of working with existing weather and climate models is that they can be quite outdated, running on old codes built by teams of people over decades.
In addition to this, these existing models have accuracy limitations, and their running on CPUs not GPUs means they are not very energy efficient. To achieve the desired result, the team needed to be able to jointly tune the weather model with the AI component together. The researchers found that rewriting the system in JAX helped to optimise this.
Insights into the long-term effects of climate change
While the increase in extreme weather events is a given due to global warming, up until now, long-term predictions of floods and storms have been difficult to model.
Moreover, Hoyer highlights that current weather forecasting lacks an understanding of the localised impact of climate change.
“This is why we need better, faster climate models,” he says. “And I am hopeful we’ll be able to use models like NeuralGCM to solve this issue.”
He continues: “I think it’s relevant for anybody who’s going to be figuring out how to deal with weather under climate change, including industry, government and individuals.”
The long-term insights have the potential to be harnessed by renewable energy providers trying to track future solar or wind capacity as well as insurance providers and those planning new infrastructure projects.
Towards AI climate modelling
Presently, while the success of the model so far is encouraging, Hoyer acknowledges that it is still very early days for using AI for weather and climate modelling, foreseeing many more steps in scaling it up to be commercially available.
He says that, currently, Google Research is focussed on enabling climate scientists to build on the work, with NeuralGCM currently available open source for non-commercial use.
Looking to the further integration of AI into climate forecasting, Hoyer adds: “I think it’s going to happen pretty quickly, probably over the next couple of years, and we’re going to see AI weather and climate models start to penetrate full-scale use cases across industry.”